Interview with Alexandre: his role as Data Scientist, and his definition and views of data sciences
“If, thanks to Data Science, at least one person has received better treatment - or even had their life saved - that’s a success in my eyes!"
Date
Share
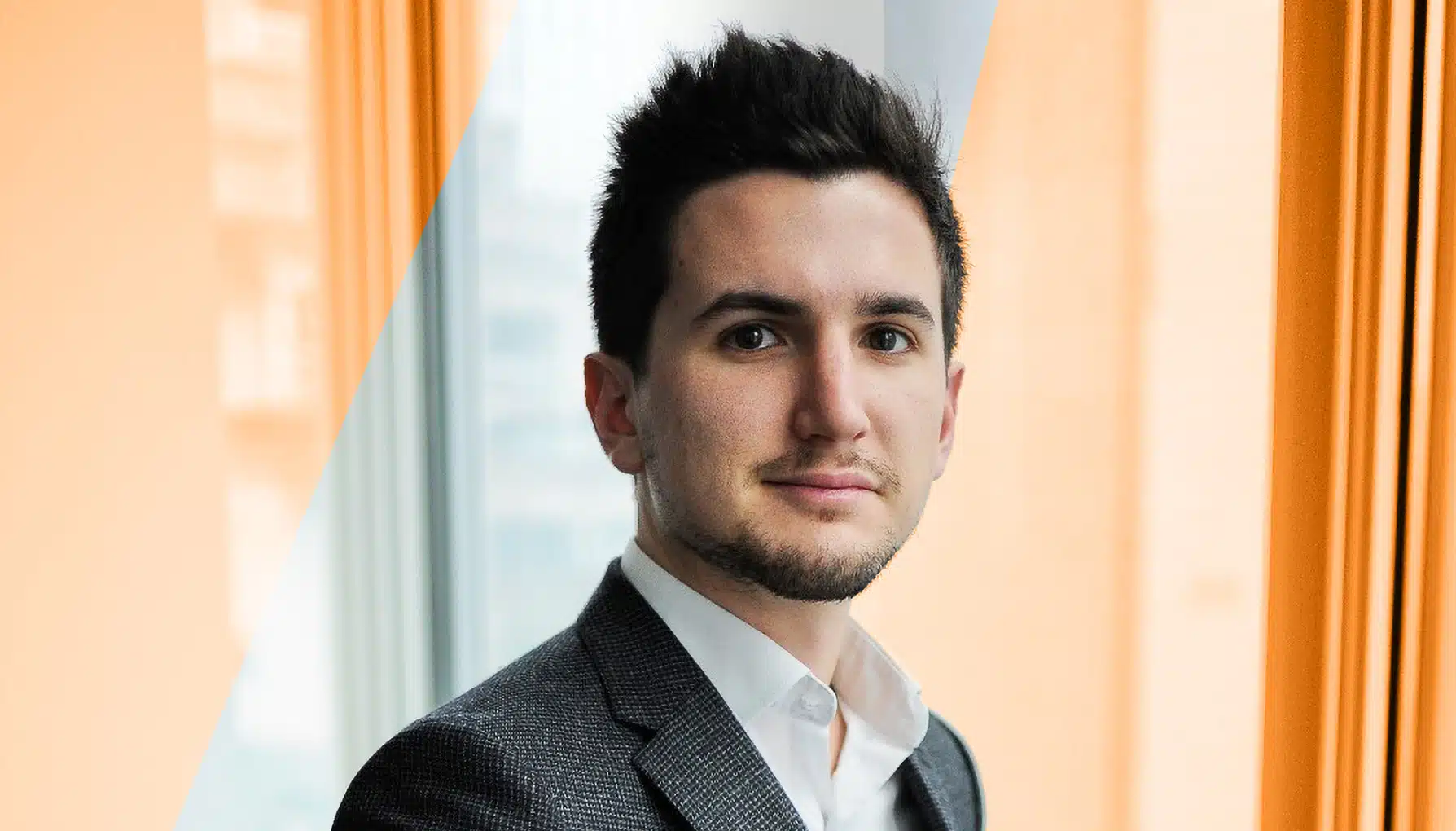
Diabeloop: So, you’re a Data Scientist at Diabeloop. How long have you been in this position, and what brought you here?
Alexandre: I joined Diabeloop around four years ago. I was drawn towards the Data Scientist career path as I’ve always had an interest in science in general, and in medicine in particular. My interest in the healthcare sector was certainly sparked after the completion of a post-graduate project in partnership with a psychiatric hospital that I took part in. During that project, I contributed to setting up a suicide risk indicator for individuals admitted into the psychiatric structure.
To do that, I used my extremely simple Machine Learning methods to propose a diagnosis support tool for psychiatrists, so that they could evaluate their patients’ suicide risks and decide on the appropriate course of action.
Having such a big impact on individuals and their lives through mathematics was truly incredible to me. If, thanks to Data Science, at least one person has received better treatment – or even had their life saved – that’s a success in my eyes!”
D: What exactly is Data Science?
A: Data Science is the use and valuation of data through mathematical and statistical methods to solve concrete problems across countless application scenarios. We’ll go into this in further detail later on, but Machine Learning is a subcategory of Data Science.
D: Have you always wanted to work in the healthcare environment?
A: No, actually – quite the contrary! I worked in consulting within a huge variety of application sectors, from mass distribution to public health, including property, transport and even the food industry – for the foie gras sector, to be specific (nope, I’m not kidding!). 😅).
Through these experiences and to fulfil my assignments, I used various techniques such as data visualisation, machine learning and algorithms in general.
D: What’s the essence of your job?
A: I add value through patient data analysis that can be used to develop new algorithms that will improve the solutions offered by Diabeloop.
D: Do you have any particular success stories from your work as a Diabeloop Data Scientist to share with us?
A: I’m delighted to have been involved in the initiative for the development of an algorithm which integrates Deep Learning and that can be used in individuals living with diabetes.
D: Data Science, Machine Learning, Deep Learning… Can you help us out with all this jargon?
A: Deep Learning is really just another way to talk about Machine Learning. This method uses mathematical/statistical techniques based on real data in order to determine a link between two variables.
Still seem a little confusing? This example might be helpful.
Imagine that we need to determine the price of a flat or house. We have two choices when it comes to our approach. We can either multiply the number of square metres by the price per square metre, or we can decide to feed an algorithm all of the property transactions made over a given period, along with a description of the properties, and we leave it to establish the link between the different variables all on its own.
This second approach uses Deep/Machine Learning.
D: What drives you on a day-to-day basis in your work?
A: First of all, I’d say it’s the technical challenge of how we can better control diabetes with as few interactions as possible from the people living with that condition. Next, it’s the interactions through feedback and conferences. Working alongside healthcare professionals and engineers, in contact with people living with chronic illness… It’s very enriching. The feedback from patients is a real motivation booster for the teams. We sometimes get lost in the smaller details, like optimisation and control issues, and forget about the bigger picture, that is, the degree to which we’re changing and improving people’s lives!
D: In your opinion, what are the key requirements for being a good Data Scientist?
A: Keeping up-to-date and well-read is essential, and you can do that by reading articles, scientific papers, conference summaries and not necessarily all relating directly to healthcare. The structure of the algorithms can be similar from one industry to the next. In healthcare, some algorithms are inspired by the world of finance, or even the mobility sector with the self-driving car.
I think that a Data Scientist can work in pretty much any sector, but to really perfect their skills and expand on certain, specific aspects, you need to be interested in a field, a company or a solution in particular. In my case, joining Diabeloop given me a better understanding of the physiological aspects of diabetes. I’d even say that I’ve learned more in terms of physiological understanding than in terms of Data Scientist skills, so to speak.
D: How would you assess your professional experience at Diabeloop?
A: Diabeloop is an organisation that really listens to its employees and gives everyone the opportunity to add their own value. It’s a really human-centred company, where employees are heard and constructive feedback is possible at all levels, regardless of role or scope. All of this is driven by a continuous quest for improvement.
The company’s hyper-growth has really struck me. When I arrived, there were only 40 of us, and now, you can’t call Diabeloop a start-up anymore. The switch from a developing company to a commercial organisation is a key and complex phase that requires a lot of adaptability. In my opinion, Diabeloop’s in a structuring phase.
D: What do you think the future holds for Data Science and its careers, in general and in relation to healthcare?
A: It’s no secret that we’re going to be faced with a boom in the quantity of data available, sooner or later. The challenge for companies will lie in knowing how to value and use this data to improve their competitive edge, and that sums up the potential added value of Data Science. When applied to healthcare, Data Science really opens up avenues with exponential opportunities. It should be known that, if used properly, Data Science will radically transform the healthcare of the future.
For me, the evolution in Data Scientists’ work goes hand-in-hand with these structuring changes. I think that it requires the creation of new positions further upstream, in terms of data management, databases, data protection, and downstream in the value chain, in terms of popularisation and evangelisation. If you ask me, one of the main points we need to tread carefully with is automation. Automating processes is a key part of our work. Yet, if we automate everything, you begin to want to be automated yourself. Also, solving complex issues requires refined and advanced skills, but it should be remembered that you can also come across more “classic” issues in Data Science, and you really need to have an interest in it.